Introduction #
In the world of online marketing, success hinges on understanding your customers. Knowing what they want, how they behave, and what influences their decisions is crucial. As a conversion rate optimization agency that has served hundreds of clients over the past 14 years, we’ve seen this truth proven time and again.
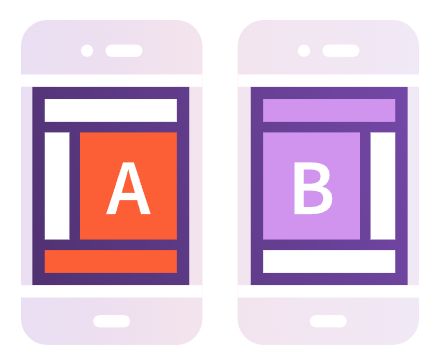
One of the most effective ways to gain this understanding is through A/B testing. Our work with clients ranging from niche Shopify stores to $100MM+ Internet 500 retailers has demonstrated that systematic testing is key to sustainable growth.
A/B testing, also known as split testing, is a method of comparing two versions of a webpage or other user experience to see which performs better. It’s a way to test changes to your webpage against the current design and determine which one produces better results.
But why is A/B testing so important?
It’s simple. A/B testing can lead to better conversion rates. That means more sales, more sign-ups, more clicks – whatever your goal might be. Through our CRO services, we’ve seen this impact firsthand – our average client achieves a 50-150x ROI from their testing program.
A/B testing allows you to make more out of your existing traffic. While the cost of acquiring new customers is rising, A/B testing allows you to increase revenue without having to bring in more visitors. For instance, one of our jewelry ecommerce clients achieved $14 million in incremental revenue through strategic testing alone.
This comprehensive guide is designed to help ecommerce, SaaS and B2B business leaders understand the nuances of A/B testing. Drawing from our experience of over 6,000 successful tests, we’ll provide a deep dive into the methodology, importance, and implementation of A/B testing for maximum conversion rates.
We’ll cover everything from formulating a hypothesis, selecting variables, creating variations, to analyzing results. We’ll also delve into the tools and technologies that can aid in A/B testing, and how to integrate them with your ecommerce platform.
Moreover, we’ll discuss common pitfalls to avoid and ethical considerations to keep in mind when conducting A/B tests, based on real scenarios we’ve encountered in our testing programs.
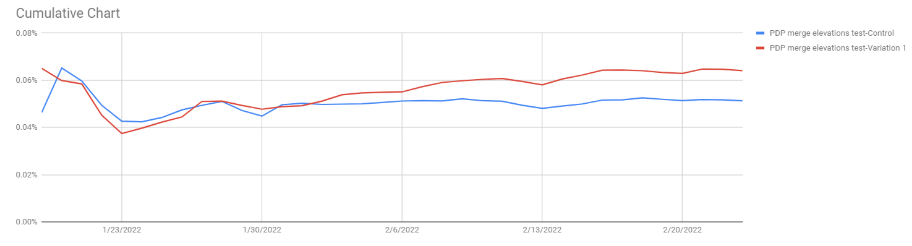
Chart shows an example AB Test cumulative conversion lift
Real-world examples from our client portfolio will illustrate the power of A/B testing in action. We’ll explore how companies across various industries – from jewelry and medical supplies to house plans and graduation regalia – have achieved significant improvements through systematic testing.
We’ll also discuss the importance of building a culture of continuous improvement and experimentation within your organization, drawing from our experience helping clients establish successful internal testing programs.
Whether you’re new to A/B testing or looking to refine your approach, this guide combines academic knowledge with practical insights from years of hands-on experience in conversion rate optimization.
Let’s dive in and start optimizing for better conversions!
Understanding A/B Testing and Its Importance #
A/B testing is a powerful tool in the arsenal of any online marketer. Through our work with hundreds of clients, we’ve seen it provide a structured way to make data-driven decisions that directly impact the bottom line.
By testing different variations, companies can learn what resonates best with their audience. For example, when one of our house plans clients tested the integration of floor plan elevations into their main product thumbnail area, they saw an 18% increase in revenue per user and a 25% increase in transaction conversion rate:
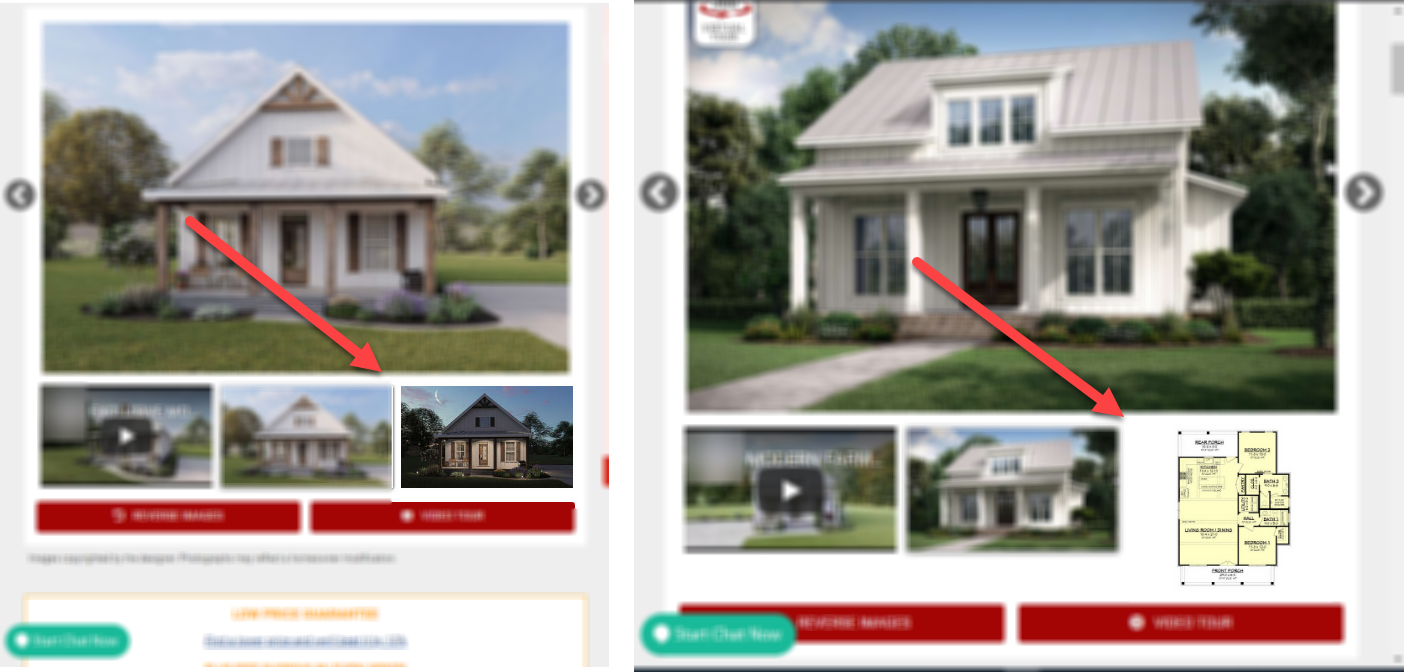
Control and winning test versions
The primary purpose of A/B testing is to optimize website performance. This could mean different things depending on your goals. For some of our clients, it means increasing sales – like our medical supplies client who achieved a 10% rise in revenues per user. For others, it might be boosting email sign-ups or improving lead generation, as we’ve seen with our B2B clients.
The beauty of A/B testing lies in its simplicity and effectiveness. It boils down complex decisions to straightforward comparisons. This makes it easier to refine website elements like headlines, images, call-to-action buttons, and more. Our testing history shows that even small changes can have significant impact – one client saw a 7.4% conversion rate increase simply by optimizing their cart page fine print.
What is A/B Testing? #
A/B testing is a comparative experiment between two or more variations. The process involves creating two versions: Version A, the control, and Version B, the variant. Users are split between these two versions to see which yields a better outcome.
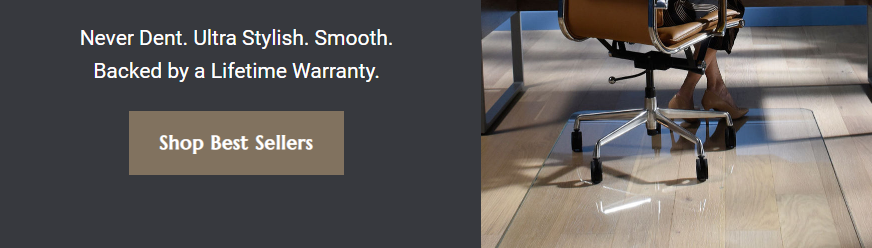
AB test example: control
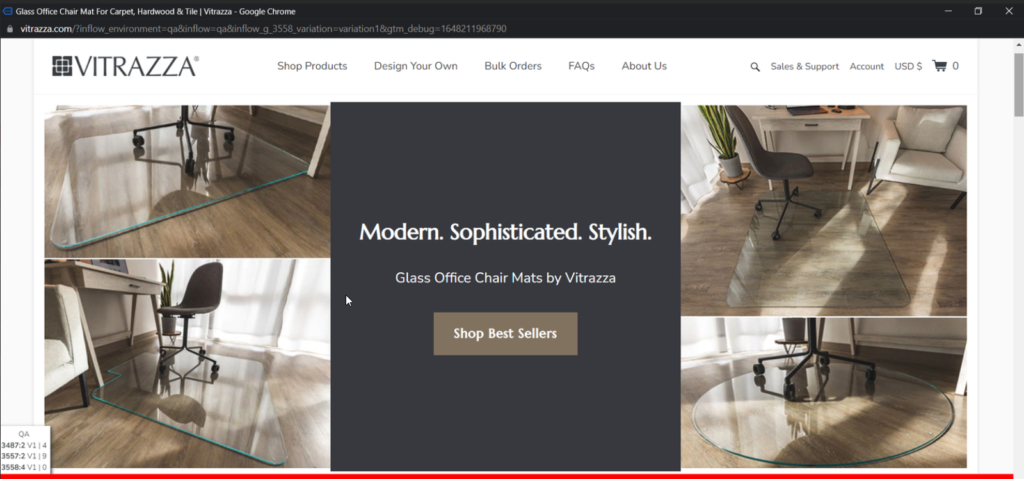
AB test example: winning test version
Consider a real example: A luxury glass mat company tested two different approaches to their value proposition:
- Control (Version A): “Glass Office Chair Mats by Vitrazza/Never Dent. Ultra Stylish. Smooth.” with single hero image
- Variation (Version B): “Modern. Sophisticated. Stylish./Glass Office Chair Mats by Vitrazza” with multiple aspirational images
This simple test led to a 9.3% increase in conversion rates, demonstrating how changes in messaging and imagery can have significant impact on how users respond to your brand.
The goal of any A/B test is to compare metrics such as click-through rates, conversion rates, or other key performance indicators. The key is identifying which metrics matter most for your specific business objectives, whether that’s lead generation, sales, sign-ups, or engagement.
Why A/B Testing is Crucial for Success #
In today’s digital landscape, businesses need data-driven methods to improve their user experience and conversion rates. Systematic testing makes the difference between guessing what works and knowing what works.
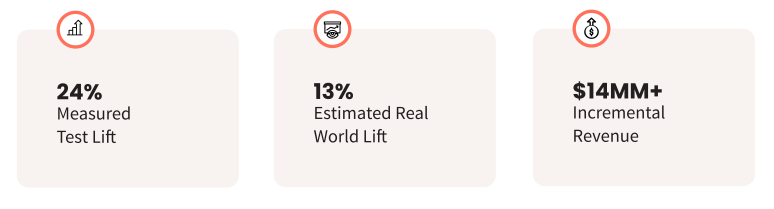
AB Testing program results for high traffic jewelry ecommerce client
A/B testing provides the data needed to make informed decisions. One major retailer demonstrated this by achieving over $14 million in incremental revenue through systematic testing of their user experience. Through carefully planned tests of their product pages, navigation, and checkout process, they were able to improve not just conversion rates but also average order value and customer satisfaction.
Testing reveals what truly resonates with your audience. The insights gained can inform decisions across your entire digital presence – from homepage messaging to checkout flow, email campaigns to social media strategy. Every touchpoint presents an opportunity for optimization and improvement.
The return on investment from A/B testing can be remarkable. While many marketing initiatives require continuous investment, improvements from successful tests continue delivering returns long after implementation. This compounds over time as you build on each success, creating an ever-improving user experience.
Overall, A/B testing is a crucial component for any business seeking to improve their digital presence. In today’s data-driven landscape, it’s no longer optional – it’s a fundamental requirement for making informed decisions about your user experience.
The Scientific Method of A/B Testing #
A/B testing is grounded in scientific principles. It relies on a specific methodology to ensure reliable outcomes. At its core, A/B testing involves hypothesis formulation, variable selection, and analyzing results.
This structured approach minimizes biases and maximizes the validity of results. It helps pinpoint the exact factors driving behavior changes.
When conducting A/B testing, it’s important to apply the scientific method consistently. This increases confidence in the decisions driven by your test outcomes.
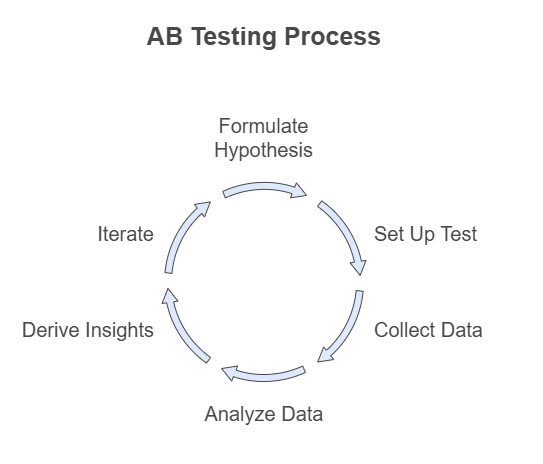
An iterative A/B testing framework showing the cyclical process from hypothesis formation through insights gathering, demonstrating how each test leads to new hypotheses and continuous optimization.
Formulating a Hypothesis
Every A/B test begins with a strong hypothesis. This hypothesis stems from your understanding of current performance and user behavior insights.
A good hypothesis is both specific and measurable. For instance, “Adding customer testimonials to product pages will increase purchase conversion rates by 15% by building trust and reducing purchase anxiety.” Note how this example includes:
- The proposed change (adding testimonials)
- The specific implementation (on product pages)
- The expected outcome (15% increase in conversions)
- The reasoning (building trust and reducing anxiety)
Selecting Variables and Creating Variations
Selecting the right variables is essential in A/B testing. Variables are the elements you plan to alter to improve performance. They could include text, visuals, layout, or functionality.
It’s crucial to test one variable at a time to isolate its effect on user behavior. This clarity ensures the results clearly reflect which change prompted differences.
Creating variations involves developing alternative versions of the chosen variables. Ensure these variations are distinct and adhere to your original hypothesis.
Understanding Control and Treatment Groups
A/B testing involves splitting your audience into two groups. The control group sees the original version, while the treatment group views the variation.
This setup is pivotal. It allows you to measure the impact of changes in real-world conditions without losing historical data.
Having a clear differentiation between control and treatment groups helps maintain test integrity. This ensures accurate measurement of changes from the tested variable.
Determining Sample Size and Test Duration
The success of an A/B test is contingent upon an adequate sample size. This ensures the results are statistically meaningful.
Calculating sample size involves considering the expected effect size and variability in your data. Using a statistically significant test calculator simplifies this task.
Test duration also plays a vital role. Tests must run long enough to collect sufficient data across different days and traffic patterns. Balancing sample size with test duration provides robust, actionable insights.
By following these steps, businesses can leverage the scientific method in A/B testing to make data-driven decisions that drive sustainable growth.
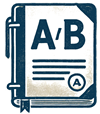
Field Notes: Practical Guidelines for Test Setup #
After thousands of tests, certain practical rules consistently prove their worth. Let’s explore the key factors that ensure reliable test results.
Test Duration Guidelines
Test duration directly impacts result validity. Always run tests for two full weeks minimum – this isn’t arbitrary. User behavior varies significantly throughout the week, with weekend visitors showing different patterns from weekday users. Friday shoppers behave differently from Monday browsers, and morning visitors often have distinct behaviors from evening visitors. Two weeks of testing helps account for these natural variations.
Sample Size Requirements
For statistically valid results, aim for at least 100 conversions per variation. When working with multiple segments:
- Each segment needs its own 100-conversion minimum
- Low-traffic sites may need extended durations
- Monitor conversion accumulation rates
Seasonal Considerations
Timing can significantly impact test results. Consider these key factors:
- Avoid major holidays unless testing holiday-specific changes
- Account for paycheck cycles in relevant industries
- Run important tests twice across different seasons
Quality Assurance Process
Quality assurance makes or breaks a test. Before launch, thoroughly check your test implementation:
- Test across all major browsers
- Verify tracking functionality
- Monitor daily metrics for anomalies
Document external factors that might influence results, such as promotional campaigns or marketing activities. These records prove invaluable when analyzing test outcomes.
These guidelines emerge from years of testing experience. Following them helps avoid common pitfalls and ensures your results are both reliable and actionable.
Setting Up Your First A/B Test #
Embarking on your first A/B test can seem overwhelming. However, following a structured process can simplify it greatly.
Before starting, define clear objectives. Know what you aim to learn from the test and how it aligns with your business goals.
Step-by-Step A/B Testing Tutorial
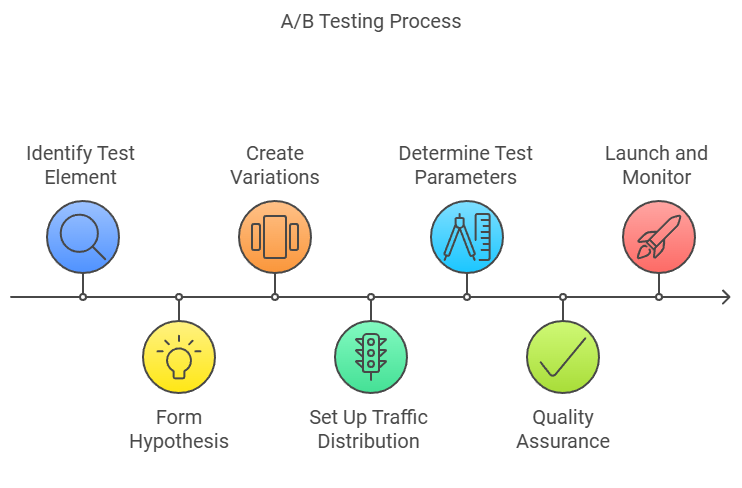
Visualizing the 7-step A/B testing process, from identifying test elements through launch and monitoring
1. Identify Your Test Element
Begin by identifying what you want to test on your website. While you might be tempted to change everything at once, focus on one key element. Common test elements include product page layouts, checkout flows, navigation structures, and value propositions. Trust signals and social proof elements are also excellent candidates for initial tests.
2. Form Your Hypothesis
A strong hypothesis forms the foundation of your test. It should clearly state what you’re changing and why you expect it to make a difference. For example: “Adding verified purchase badges to product reviews will increase purchase conversion rates by reducing buyer hesitation and building trust. Success will be measured by comparing purchase rates between variations.”
3. Create Your Variations
Your test needs two versions: the control (your current version) and the variation (your proposed change). Keep variations focused on testing one major change at a time – this ensures clear results and makes analysis straightforward.
4. Set Up Traffic Distribution
Traffic distribution determines how visitors experience your test. For standard A/B tests, use a 50/50 split between control and variation. When testing major changes, consider using weighted distribution to limit exposure. Multiple variations should receive equal splits unless you have specific reasons for different weightings.
5. Determine Test Parameters
Before launch, establish your test framework:
- Duration: Plan for minimum two weeks
- Sample size: Calculate based on your traffic
- Success metrics: Define primary and secondary goals
- Tracking setup: Ensure proper measurement
6. Quality Assurance
QA is crucial for valid results. Before launch, verify that:
- Both versions render correctly across devices
- All tracking is working properly
- User paths function as intended
- No other tests will conflict
7. Launch and Monitor
Once your test is live, maintain active oversight. Monitor performance daily, but resist the urge to peek at results too early. Document any external factors that might impact your test, such as marketing campaigns or seasonal events.
Remember that your first test sets the foundation for your testing program. Take time to do it right, and use the learnings to improve future tests.
Critical Considerations #
Traffic Requirements
Sufficient traffic is fundamental to meaningful test results. Start by calculating your required sample size based on expected effect – this will vary depending on your baseline conversion rate. For example, if your site converts at 2%, you’ll need more traffic than a site converting at 10% to detect the same percentage improvement.
Consider these factors when planning your test duration:
- Baseline conversion rates
- Expected effect size
- Seasonal traffic patterns
- Typical daily fluctuations
Technical Setup Challenges
Most testing challenges stem from technical implementation issues. The flicker effect, where users briefly see the original page before the variation loads, can significantly impact results. Similarly, mobile responsiveness problems often go unnoticed without thorough cross-device testing.
Two other common pitfalls require careful attention:
- Tracking configuration errors that miscount conversions
- Cache-related problems that serve incorrect variations
Determining Test Completion
Knowing when to conclude your test is as important as knowing how to start it. While achieving statistical significance is crucial, it’s just one of several completion criteria. Your test should run its full planned duration even if significance is reached early – this helps account for any weekly patterns in user behavior.
Always verify these conditions before concluding:
- Statistical significance achieved
- Minimum sample size reached
- Full test duration completed
- No major external factors impacting results
Remember, proper setup is crucial for reliable results. Take the time to plan and execute your test carefully – rushing the process often leads to inconclusive or misleading outcomes.
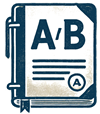
Field Notes: Common AB Test Setup Pitfalls #
The Critical Importance of QA
In our years of running thousands of tests, we’ve learned that Quality Assurance is the most overlooked yet crucial aspect of test setup. We’ve seen countless “losing” tests that were actually winners masked by technical issues.
Here’s what we’ve learned to watch for:
Browser compatibility is particularly tricky. We often find variations that perform beautifully in Chrome but fail in Safari or Edge. That’s why we always track results by browser – we’ve seen tests that appeared to be losing overall but were actually winning in 4 out of 5 browsers.
For thorough QA, we recommend:
- Using cross-browser testing tools (we use LambdaTest; other alternatives include CrossBrowserTesting, BrowserStack, and Sauce Labs)
- Testing across all major operating systems
- Verifying both desktop and mobile experiences
KPI Identification and Tracking
We’ve seen many testing programs focus solely on conversion rate, missing crucial insights. Through our experience, we’ve identified several critical metrics that tell the complete story.
When we set up a test, we always track:
- New element engagement (clicks, hovers, form fills)
- Existing element impact (especially for elements we de-emphasize)
- User flow changes
- Time on page
- Micro-conversions
One of our most important learnings has been the value of segment-specific KPIs. We’ve seen tests that look neutral overall but show dramatic improvements for specific user segments. That’s why we always track metrics separately for new versus returning users, and often by traffic source as well.
Before launching any test, we establish baseline metrics across all these dimensions. We’ve found this comprehensive approach essential for truly understanding test results – more than once, we’ve uncovered winning variations that would have been missed with simpler tracking.
Tools and Technologies for A/B Testing and Feature Management #
The landscape of testing and feature rollout tools has evolved significantly. While A/B testing and feature flagging were once distinct categories, modern platforms increasingly offer overlapping capabilities.
Understanding A/B Testing vs. Feature Flagging
A/B Testing Platforms
Traditional A/B testing platforms focus on optimizing user experiences and measuring their impact. These tools excel at testing marketing changes and UX improvements through visual editors, providing statistical analysis and user segmentation capabilities.
Feature Flagging Systems
Feature flags serve a different primary purpose: controlling feature rollouts and managing functionality. They enable gradual releases to users and provide kill switches for problematic features, offering granular control over how and when users experience new functionality.
The Growing Overlap
Modern platforms increasingly blend these capabilities, creating a more comprehensive approach to experimentation. Feature flags now power sophisticated A/B tests, while testing platforms incorporate feature management capabilities. This convergence offers organizations more flexibility in how they approach both testing and feature releases.
Many organizations use both tools complementarily:
- Technical teams use feature flags for gradual rollouts
- Marketing teams leverage A/B testing for UX optimization
- Product teams combine both for complex experiments
Platform Categories
Enterprise Solutions
Enterprise platforms offer comprehensive testing capabilities suited for large organizations. Adobe Target provides deep integration with Adobe Analytics, while Optimizely delivers advanced experimentation features. Other major players include Dynamic Yield for personalization and SiteSpect for server-side testing capabilities.
Mid-Market Solutions
Growing businesses often find their sweet spot with platforms like VWO (Visual Website Optimizer) or Convert. These tools balance functionality with usability, offering robust testing capabilities without overwhelming complexity. Kameleoon and AB Tasty provide similar capabilities with regional strengths.
Free and Open Source Options
Several powerful options exist for teams getting started or those preferring open-source solutions.
At ConversionTeam, we’ve developed a free AB testing tool called Nantu, which represents a significant advancement in accessible testing. After powering over 6,000 successful tests for hundreds of clients, we’ve made our battle-tested platform available as a free, open-source solution. Nantu eliminates the traditional cost barriers of premium A/B testing platforms while providing enterprise-grade capabilities:
- Seamless integration with Google Tag Manager and other popular tag managers
- Advanced variation management and precise user targeting
- Comprehensive QA tools including preview modes and anti-flicker protection
- Built-in Microsoft Clarity integration for heatmaps and session recordings
- Production-ready codebase proven across thousands of implementations
PostHog and Growth Book provide additional open-source alternatives, each with unique strengths in analytics and feature flagging respectively. Google Optimize used to be the best option here, but was discontinued.
Selecting the Right Tool
Tool selection should align with your team’s capabilities and needs. Consider these key areas:
Integration Capabilities
Your testing platform must work seamlessly with your existing tech stack. Consider compatibility with:
- Analytics platforms
- Tag management systems
- Content management systems
- Development workflows
Technical Requirements
Pay particular attention to:
- Page load impact
- Flicker prevention methods
- Cross-browser compatibility
- Mobile responsiveness
Implementation Process
A successful implementation typically involves:
- Setting up tag manager integration
- Configuring tracking events
- Establishing QA procedures
- Validating data collection
Remember that the right tool balances your technical needs, team capabilities, and budget constraints. Focus on platforms that offer the features you’ll actually use rather than getting distracted by capabilities you may never need.
Last edited just now
AB Test Implementation Best Practices #
Successful A/B testing implementation requires careful attention to both technical setup and ongoing maintenance. Let’s explore the key areas that ensure smooth test operation.
Initial Setup
The foundation of your testing program starts with proper implementation. Begin with tag manager deployment – this provides flexibility and control over your testing setup. Once your tag manager is in place, focus on establishing proper tracking events that align with your business goals. User targeting configuration comes next, followed by thorough QA procedures to ensure everything works as intended.
Technical Considerations
Performance is crucial for valid test results. Focus on four key areas:
- Page Load Impact: Minimize the effect on page speed
- Flicker Prevention: Ensure smooth variation delivery
- Cross-Browser Testing: Verify functionality across browsers
- Mobile Responsiveness: Maintain consistent experience across devices
Common Integration Challenges
Performance Optimization
Testing tools can impact site performance in several ways. Your implementation should address:
- Code efficiency in variation delivery
- Strategic asset loading
- Proper cache management
The choice between server-side and client-side implementation will depend on your specific needs and technical infrastructure.
Tracking Implementation
Reliable data collection forms the backbone of your testing program. Set up your tracking in phases:
- Configure event tracking to capture user interactions
- Establish clear goals aligned with business objectives
- Set up custom metrics for deeper insights
- Validate data collection across all variations
Remember, successful implementation balances technical efficiency with reliable data collection. Take time to get these fundamentals right – they’ll save you countless hours of troubleshooting later.
Analyzing A/B Testing Results #
Once your A/B test concludes, diving deep into the results is crucial. This analysis guides future business decisions and helps build a foundation of knowledge for subsequent tests.
Analytics Platform Integration
Most A/B testing platforms offer integrated reporting tools, but we’ve found it’s almost always better to push your test data directly into your existing analytics platform, whether that’s Google Analytics, Adobe Analytics, Matomo, Mixpanel, or whatever platform you’re using. This approach offers several advantages:
- Analyze test data alongside your regular metrics
- Leverage existing segments and goals
- Maintain consistent reporting
- Access historical context
- Use familiar tools and interfaces
Our free AB testing tool Nantu follows this philosophy by passing testing data directly to your analytics platform, where it’s governed by your existing privacy settings and policies.
Understanding Statistical Significance
Statistical significance tells us whether results are likely real or random chance. While a 95% confidence level is standard in A/B testing, meaning you’re 95% certain the results are real, statistical significance alone doesn’t tell the whole story.
Your analysis should consider several key factors:
- Sample size adequacy
- Effect size (magnitude of change)
- Test duration and seasonality
- External factors affecting results
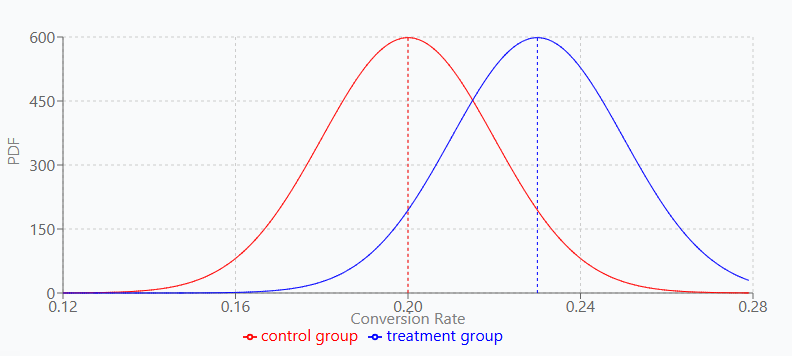
Probability density plot comparing conversion rates between control (red) and treatment (blue) groups, showing a notable shift from 20% to 23% conversion rate with the tested changes.
Working with Test Calculators
Modern testing platforms include statistical calculators that simplify significance evaluation. To use these effectively, you’ll need to gather four essential pieces of information:
- Number of visitors per variation
- Number of conversions per variation
- Original conversion rate
- Desired confidence level
Understanding these components helps you make informed decisions about test completion and validity.
Interpreting Test Results
Result interpretation requires a holistic view of your data. Start with your primary metrics – conversion rate changes, revenue impact, and average order value shifts. Then examine secondary metrics like time on site and pages per session to understand the broader impact of your changes.
Key Metrics to Monitor
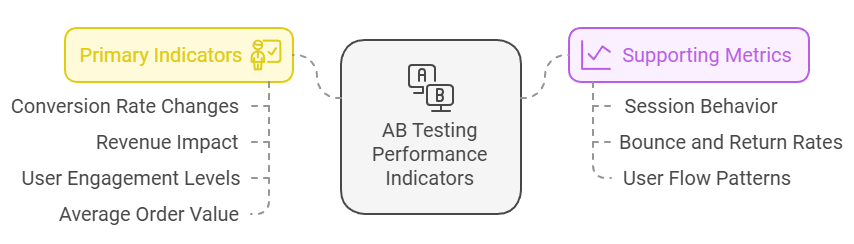
When evaluating test outcomes, we track two key categories of metrics. The primary indicators directly measure business impact through conversion rates, revenue changes, user engagement, and average order values. These are supported by behavioral metrics that provide context about how users interact with the site, including session data, bounce rates, and navigation patterns through the conversion funnel.
Segment Analysis
Different user groups often respond differently to changes. Always analyze results across:
- New vs. returning visitors
- Traffic sources
- Device types
- Geographic locations

Field Notes: Analytics Integration Best Practices #
We’ve found that integrating test data with existing analytics platforms like google analytics provides the most reliable and actionable insights. Your primary analytics platform already contains rich historical data and segment definitions – use this to your advantage. When setting up your testing program, prioritize tools that can seamlessly integrate with your analytics platform rather than relying on standalone reporting tools.
Common Analysis Pitfalls #
In our experience, certain mistakes consistently undermine test analysis. The most critical error is stopping tests too early – it’s tempting to call a test when you see strong early results, but this often leads to incorrect conclusions. Equally important is the tendency to focus on overall metrics while missing crucial segment-level insights that could reveal important patterns in user behavior.
Secondary metrics often tell an important part of the story that primary metrics miss. For instance, a test might show neutral conversion rates but significantly impact user engagement or return visit rates. External factors like marketing campaigns, seasonality, or competitor actions can also skew results if not properly considered in your analysis.
Post-Analysis Process
After completing your analysis, the real work of applying your learnings begins. Start by documenting your findings thoroughly – not just the numbers, but the insights and hypotheses they generate. Share these insights with stakeholders in a way that connects test results to business objectives.
For successful tests, develop a clear implementation plan that considers technical requirements and potential risks. Use your learnings to generate new test hypotheses, building on what worked and addressing what didn’t. Finally, update your testing roadmap to reflect your new understanding of user behavior and preferences.
This cyclical process of testing, learning, and refining ensures your optimization program continues to deliver value over time.
Documentation Best Practices #
Comprehensive documentation builds valuable institutional knowledge. Your test documentation should include:
Essential Elements:
- Original hypothesis and rationale
- Test setup and technical details
- Key metrics and segment findings
- Visual evidence (screenshots)
- Recommendations for future testing
Maintain organized, accessible documentation to inform future testing strategies and ensure your testing program continues to improve over time.
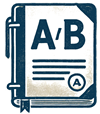
Field Notes: Essential Result Breakdowns #
WE think every A/B test should include five critical data breakdowns in its analysis. These segments consistently prove valuable for validating results and uncovering deeper insights.
Standard Breakdowns to Track
Overall Results Track the complete dataset first to understand general performance and ensure statistical validity.
New vs. Returning Users These segments often behave quite differently and may require distinct optimization strategies. Breaking out these results can reveal opportunities for personalization.
Channel Performance Different traffic sources (paid, organic, direct, email) can respond uniquely to site changes. Understanding channel-specific impacts helps validate test results and informs future marketing decisions.
Browser Breakdown This technical breakdown is crucial for catching implementation issues. A test showing poor results overall might actually be winning in most browsers but failing in one due to technical issues.
Device Type Mobile, desktop, and tablet users navigate differently and may respond uniquely to changes. Always verify your test performs well across all devices.
Understanding these breakdowns helps validate both the technical implementation and business impact of your tests.
Common Pitfalls and Ethical Considerations in A/B Testing #
A/B testing is powerful, but it has its challenges. Understanding common pitfalls and ethical considerations helps ensure your testing program’s success and integrity.
Avoiding Common Mistakes
Clear Goals and Objectives
Running tests without defined objectives wastes resources and leads to misleading conclusions. Every test should start with clear business objectives that specify what metrics you aim to improve and how success will be measured. These objectives must align with broader business goals to ensure your testing program delivers meaningful value.
Test Design Fundamentals
Poor test design can undermine even the best optimization programs. The most common design mistakes stem from trying to test too many variables simultaneously, which makes it impossible to determine what actually drove results. Seasonal factors often confound test results when not properly accounted for, while running multiple conflicting tests can invalidate all your results at once.
Sample size is particularly crucial – insufficient data leads to unreliable conclusions. Before launching any test, calculate your required sample size based on your baseline conversion rate and the minimum improvement you need to detect.
Result Interpretation
Accurate interpretation requires understanding both statistics and context. Two critical mistakes often occur: stopping tests too early based on promising initial results, and making decisions without considering segment-level insights. External factors like marketing campaigns or competitor actions can significantly impact results, so document any unusual circumstances during your test period.
Ethical Testing Practices
Privacy and Data Protection
Testing must respect user privacy and data protection laws, particularly GDPR requirements. Develop clear practices for:
- Data collection and storage
- Cookie consent management
- User privacy protection
- Security protocols
Transparency in Testing
Building trust requires honesty about testing practices. Start by updating privacy policies to reflect your testing activities. Consider how and when to notify users about ongoing tests, while maintaining transparency about data handling practices. Always respect user preferences regarding participation in tests.
Fair Treatment and Accessibility
Testing should improve experiences for all users, not just some. Consider these key principles:
- Test impacts across different user groups
- Maintain accessibility standards
- Monitor for unintended consequences
- Prepare rollback procedures
A/B Testing in Action: Real-World Examples #
Understanding how A/B testing works in practice helps illuminate its potential and challenges. Let’s explore the most common testing categories and principles that drive successful optimization programs.
Testing Categories
User Experience Optimization
User experience testing focuses on how visitors interact with your site. Navigation structures often present significant opportunities for improvement, as do search functionality and form design. The key is identifying friction points in your user journey and systematically testing solutions to resolve them.
Content and Messaging
Content testing reveals what truly resonates with your audience. Start with your value proposition – how you communicate your core benefits can dramatically impact engagement. Test variations in call-to-action text, product descriptions, and trust signals to find the most effective way to communicate with your users.
Technical Implementation
The technical side of testing requires careful attention to both functionality and performance. Begin with fundamental elements like:
- Checkout process optimization
- Site speed improvements
- Interactive feature enhancements
- Cross-device compatibility
Principles of Success
Methodological Approach
Successful testing programs share a systematic approach to experimentation. Each test should start with a well-defined hypothesis based on user data or behavioral insights. Implementation requires careful attention to detail, while proper monitoring ensures you catch any issues early. Finally, thorough analysis helps you extract maximum value from each test.
Continuous Learning
A/B testing is fundamentally a learning process. Every test, regardless of outcome, should generate insights that inform your optimization strategy. Success comes from:
- Documenting clear learnings from each test
- Building on insights systematically
- Sharing knowledge across teams
- Refining your testing strategy
Remember that A/B testing is an iterative process. Each experiment builds upon previous learnings, gradually deepening your understanding of user behavior and preferences. The key is maintaining consistent documentation and analysis practices that help you build on each success and learn from each failure.
Case Studies of Successful A/B Tests #
Real-world examples reveal the potential of A/B testing. These case studies demonstrate how systematic testing leads to significant improvements.
Jewelry Retailer: Portal Page Optimization #
Challenge: Portal pages weren’t effectively showcasing products to users.
Solution: Added top products for each section on portal pages.
Results:
- 36% increase in revenue per user
- 5.2% increase in transaction rate
- 34.8% improvement for organic traffic
Key Learning: Strategic product presentation can significantly impact user engagement and purchase decisions.
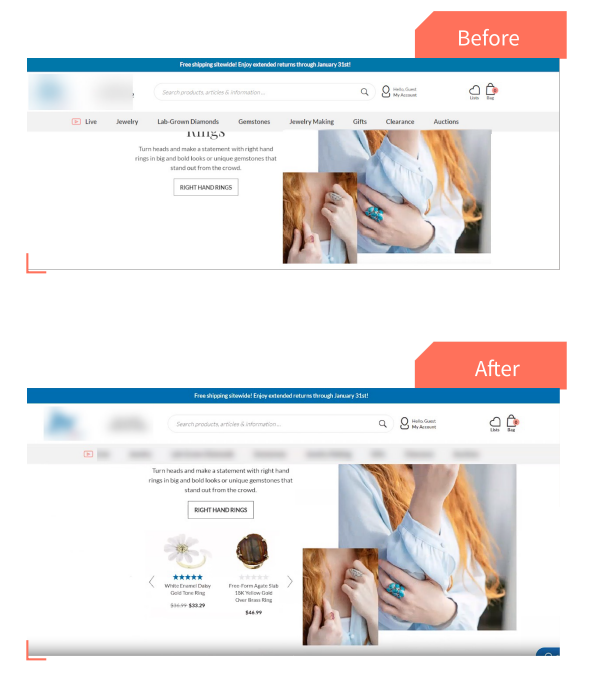
Test Version: Top products added to the page
House Plans Website: Navigation Enhancement #
Challenge: Users struggled to find floor plan information efficiently.
Solution: Integrated floor plan elevations into the main product thumbnail area.
Results:
- 25% increase in transactions
- 18% increase in revenue per user
- 28% improvement for returning users
Key Learning: Making critical information more accessible can dramatically improve conversion rates.
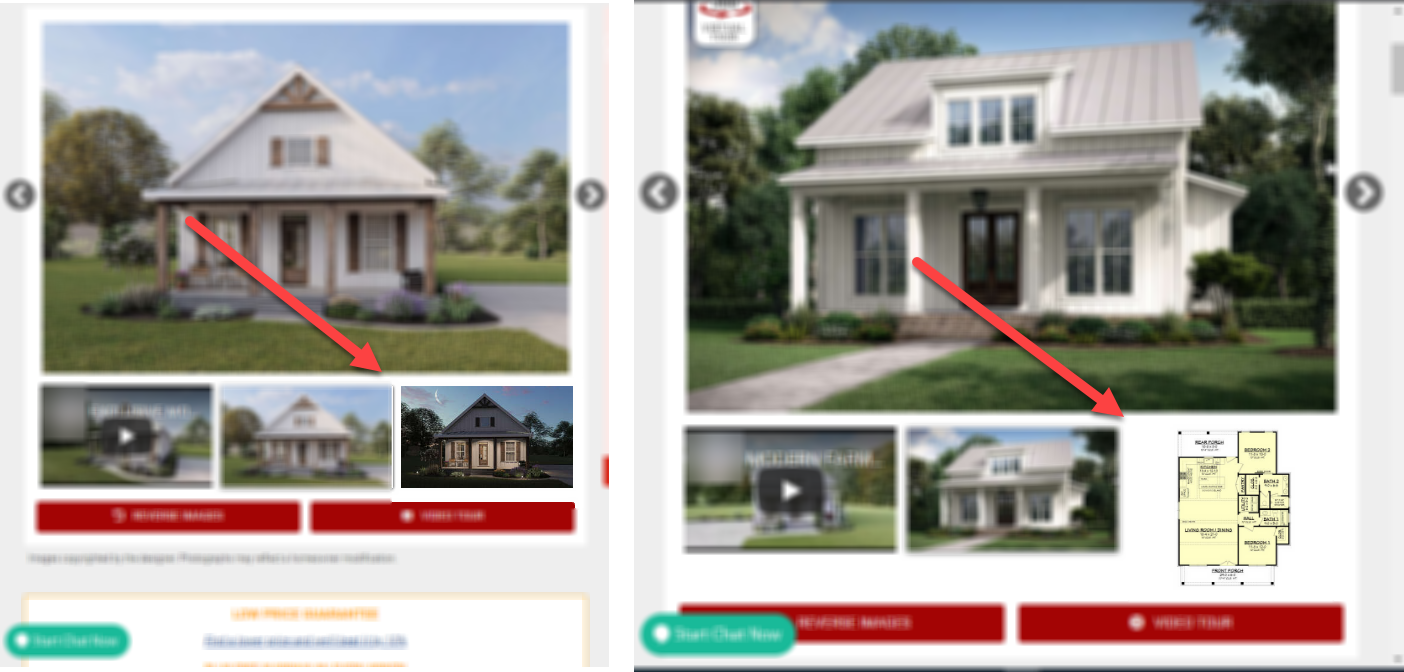
Control and test variation winner
Professional Education: Navigation Clarity #
Challenge: Professional development programs were hard to discover.
Solution: Optimized navigation labels and structure.
Results:
- 135.2% lift in conversion rate
- Significant improvement in program discovery
- Better user engagement across segments
Key Learning: Clear navigation structure directly impacts user conversion rates.
Advanced A/B Testing Strategies #
As your testing program matures, incorporating advanced methods can provide deeper insights and better results. Let’s explore sophisticated approaches that can enhance your optimization efforts.
Multivariate Testing vs. A/B Testing #
Multivariate testing represents a significant step beyond basic A/B testing. While A/B testing examines one change at a time, multivariate testing enables you to evaluate multiple elements simultaneously. This approach proves particularly valuable when optimizing complex pages or entire design systems.
Consider these key differences:
A/B Testing:
- Tests single changes
- Requires less traffic
- Provides clear, direct insights
Multivariate Testing:
- Tests multiple variables simultaneously
- Needs larger sample sizes
- Reveals interaction effects between elements
While powerful, multivariate testing demands careful consideration. You’ll need substantial traffic to achieve significant results, along with meticulous planning and analysis to derive actionable insights.

Field Notes: The Reality of Multivariate Testing #
In our experience, multivariate testing is often more trouble than it’s worth except for extremely high traffic sites & apps (ie: 1 million+ users a day). While the concept of testing multiple variables simultaneously is appealing, the practical challenges usually outweigh the benefits.
You’re almost always better off running a series of A/B or A/B/n tests than attempting one multivariate test. Here’s why: A/B tests provide clearer insights about specific changes, require less traffic to reach statistical significance, and are easier to analyze and act upon. This sequential approach also allows you to build on each learning systematically rather than trying to interpret complex interactions between multiple variables.
Even with substantial traffic, the increased complexity of multivariate testing often leads to inconclusive results or insights that are difficult to act upon. Save multivariate testing for specific scenarios where you have both massive traffic volumes and a genuine need to understand variable interactions.
Personalization and Segmentation #
Advanced testing programs often incorporate personalization and segmentation to deliver more targeted experiences. This approach goes beyond simple A/B splits to consider specific user characteristics and behaviors.
Start by segmenting users into meaningful groups based on:
- Behavior patterns
- Purchase history
- Traffic source
- Device type
Then craft personalized experiences for each segment. This targeted approach typically yields higher conversion rates because it addresses specific user needs and preferences rather than trying to find a one-size-fits-all solution.
Scaling Your Testing Program #
As your business grows, your testing program must evolve to match. Scaling involves more than just running more tests – it requires a systematic approach to expansion that balances increased testing volume with maintained quality and insights.
Start by ensuring your technical foundation can support growth. Your testing tools need to handle increased volume while maintaining reliable data collection and analysis capabilities. This might mean investing in more robust platforms or upgrading your analytics infrastructure to process larger datasets efficiently.
Strategic prioritization becomes crucial as you scale. Focus your expanded testing efforts on areas with the highest potential impact: critical conversion paths, high-traffic pages, and key revenue-driving features. This targeted approach helps maintain program efficiency while maximizing returns on your testing investment.
Automation plays a vital role in successful scaling. Look for opportunities to streamline test deployment, data collection, and reporting processes. Integration with your CRM and other business systems helps maintain a comprehensive view of your testing impact while reducing manual work.
Remember that successful scaling requires patience and systematic expansion. Build processes that can grow with your business while ensuring each test continues to provide valuable insights. Focus on creating sustainable systems rather than simply increasing test volume – quality should never be sacrificed for quantity.
Building a Culture of Continuous Improvement #
Creating a culture of continuous improvement is essential for long-term testing success. Rather than treating tests as one-off activities, successful organizations integrate testing into their core business operations. Let’s explore how to build and maintain this culture.
Key Elements of Testing Culture
Leadership Commitment
Success starts at the top. Leadership must demonstrate active commitment to the testing program through consistent resource allocation and regular involvement in testing discussions. This commitment extends beyond simple approval – leaders need to champion data-driven decisions and demonstrate patience with the testing process, understanding that meaningful results take time.
Team Development
Empowering your team is crucial for sustained success. This means providing comprehensive training and education opportunities while ensuring access to necessary tools and resources. Create clear decision-making frameworks that help team members work autonomously, and allocate sufficient time for proper analysis and learning.
Knowledge Management
Building and maintaining institutional knowledge requires systematic documentation and regular knowledge sharing. Schedule regular team reviews to discuss test results and insights. Create and maintain testing playbooks that capture best practices and learnings, ensuring this knowledge remains accessible as your team grows.
Implementing Your Testing Program
Strategic Approach
Effective testing programs require careful planning and execution. Start by:
- Aligning tests with clear business objectives
- Identifying and prioritizing high-impact opportunities
- Allocating resources strategically
- Setting realistic timelines for implementation
Review and Iteration
Establish a rhythm for your testing program that includes:
- Weekly monitoring of active tests
- Monthly analysis of results and trends
- Quarterly strategy reviews and adjustments
- Annual program evaluation and planning
Learning from Every Test
Success Analysis
When tests succeed, take time to understand why. Document specific changes that worked and analyze the reasons behind their success. Look for opportunities to apply these learnings in other areas, and plan follow-up tests to build on your success.
Turning Failure into Opportunity
Test failures provide equally valuable insights when properly analyzed. Take time to:
- Understand what didn’t work and why
- Extract actionable insights for future tests
- Refine your testing hypotheses
- Share learnings across the team
Remember that building a testing culture takes time and patience. Focus on creating sustainable processes that support long-term improvement rather than chasing quick wins. Your goal is to build a program that consistently delivers value while continuously evolving based on new insights and learnings.
Conclusion and Next Steps #
A/B testing is a powerful tool for improving digital experiences and business outcomes. Through systematic testing and analysis, organizations can make data-driven decisions that drive sustainable growth.
Getting Started
Begin your testing journey with these key steps:
- Define clear objectives for your testing program
- Choose appropriate tools for your needs
- Start with high-impact, low-complexity tests
- Build a process for documentation and learning
For hands-on guidance in starting your testing program, consider getting a free Quick Wins CRO audit.
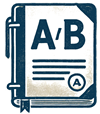
Field Notes: The Value of Experience #
A/B testing is complex, with many moving parts and potential pitfalls. While this guide provides a foundation for understanding testing principles, experience matters significantly in practice. Every industry, website, and user base presents unique challenges that often require specialized knowledge to navigate effectively.
Whether you’re just starting out or looking to enhance your existing program, we’re here to help. Get in touch to discuss your testing goals or learn how we can build a comprehensive testing program for your business. You can also reach us at (720) 443-1052.
Appendix #
A/B Testing Checklist #
Test Planning
- Define clear business objectives
- Form specific hypothesis
- Identify primary and secondary metrics
- Determine required sample size
- Plan test duration (minimum two weeks)
- Document technical requirements
Test Setup
- Implement tracking correctly
- Set up proper segmentation
- Configure analytics tools
- Perform cross-browser testing
- Check mobile responsiveness
- Validate tracking setup
Test Monitoring
- Monitor daily metrics
- Check for technical issues
- Track external factors
- Document any anomalies
- Watch segment performance
Glossary of A/B Testing Terms #
A/B Test A randomized experiment comparing two variants of a webpage or app.
Confidence Level Statistical measure indicating result reliability, typically set at 95%.
Control The original version in an A/B test.
Conversion Rate Percentage of visitors who complete a desired action.
Effect Size The magnitude of difference between control and variation.
Hypothesis A testable prediction about the impact of changes.
Sample Size Number of visitors needed for statistically valid results.
Segmentation Division of traffic based on user characteristics.
Statistical Significance Likelihood that results are not due to random chance.
Variation The modified version being tested against the control.
Additional Resources #
Tools and Platforms
Further Reading
- The Needle Blog
- Ecommerce Product Page Best Practices: The perfect PDP
- The Ultimate Black Friday E-commerce Optimization Checklist
- Ecommerce Product Listing Page Best Practices: Maximizing Conversions for the Perfect PLP
Start your testing journey with a solid foundation. Remember that successful A/B testing is an ongoing process of learning and improvement, not a one-time effort.